
GNSS Integrity for Autonomous Driving
The automotive world is barrelling towards an autonomous future driven by the hope of dramatically safer roads and the lure of stress-free commutes and driverless transport services. The technology behind this development encompasses RADAR, Machine Vision, LiDAR and high-definition maps coupled with machine learning and artificial intelligence to provide collision avoidance, landmark-based positioning and environmental awareness. This suite of technologies will meet most requirements to guide a vehicle safely through the road network, but it is not enough. A reliable, high-precision source of absolute position is also needed1, and Global Navigation Satellite Systems (GNSS) based positioning is the only globally available technology that can provide it. (GNSS includes the original GPS, GLONASS, Galileo, and BeiDou. Multi-constellation receiver chips are readily available.)
GNSS is needed to unambiguously identify the road and road segment that the vehicle is on. This is essential as it determines what level of autonomy is permitted and which threats are present. Fig. 1 illustrates road conditions where such autonomy will likely be permitted initially. The other sensor subsystems often require recalibration on the fly and GNSS is an ideal independent source of position, time and velocity information for this. In addition, GNSS is an independent cross-check for landmark-based positioning. Simultaneous Location And Mapping (SLAM) is a scheme for crowd-sourced map updating concurrent with the maps being used for landmark-based positioning. GNSS can make SLAM far more reliable and efficient by providing an independent location source for the map updating. GNSS can also potentially be used for lane identification. Finally, unlike vision, for example, GNSS is largely unaffected by sleet, snow, smoke, rain, fog etc.
Figure 1 – Examples of Highway Conditions Where Stage 3 Autonomy Will Likely Be Permitted Initially
GNSS in automotive environments suffers from a number of vulnerabilities. GNSS signal tracking is not continuous even on the highway. The signals are interrupted by trees, buildings, bridges and tunnels. The way to overcome this is to couple GNSS with Inertial Navigation Systems (INS) incorporating the use of Inertial Measuring Units (IMUs) and Wheel Speed Sensors (WSS). The GNSS is used to initialise and calibrate the INS which then provides positioning for those periods when the GNSS signals are unavailable. In more tightly coupled variants of GNSS/INS positioning systems, both GNSS and INS are used concurrently.

If GNSS is to be used for safety critical automotive applications, however, GNSS/INS positioning subsystems are required that comply with automotive functional safety (FuSa) requirements. The ISO 26262 standard places strict requirements on the way systems are designed, developed and verified. Equipment is certified to one of four levels of safety (Automotive Safety Integrity Level) – ASIL A, ASIL B, ASIL C and ASIL D, ASIL A being the lowest level. The car maker typically decides which level is required based on the way the subsystem will be used and on the safety strategy and safety goals that have been adopted.
However, FuSa is only part of the story.
It is concerned with limiting the risks associated with potential failures of hardware and software within the equipment. Another standard also comes into play – ISO/PAS 21448 deals with Safety Of The Intended Function (SOTIF). It is concerned with limiting the risks that exist even when the equipment is performing its functions perfectly. In the case of GNSS, those risks derive from failures in the satellites and the GNSS systems more broadly, large errors arising from atmospheric disturbances such as tropospheric and ionospheric storms and ionospheric scintillation and, most prevalently, large errors deriving from multipath distortion of the signals and pure reflections (Non-Line-Of-Sight – NLOS). The GNSS Integrity problem we are discussing in this article is posed by the SOTIF requirements.
Note that further SOTIF threats arise when sensor measurements (e.g. from inertial measurement units and wheel speed sensors) are fused into the solution. This is because such sensors, even when working perfectly, are affected by temperature, vibration, wheel slip, tyre wear, tyre deformation and so on. Though important these are outside the scope of this article.
GET CW JOURNAL ARTICLES STRAIGHT TO YOUR INBOX Subscribe now |

The GNSS integrity problem was solved for aviation many years ago.
Researchers came up with the concept of the Protection Level (PL) which is a dynamic statistical upper limit on the positioning error. The probability that the Positioning Error (PE) could exceed the PL without a timely alert must be guaranteed to be below a very low number such as 10-8/operation. This probability is known as the Integrity Risk (IR). In this context, an ‘operation’ is a manoeuvre, such as landing approach, landing or take-off or part of a phase of flight such as en-route and may last for many minutes at a time. Typically, in aviation, the positioning system provides a Vertical Protection Level (VPL) and a Horizontal Protection Level (HPL) with each position update.
The PL is used by the system in conjunction with an Alert Limit (AL). The value of the alert limit depends on the current use of the position by the system and hence the AL may change from time to time. If the PL exceeds the AL then this is an indication that the risk posed by possible positioning errors is too great for the operation and the system should discontinue using the positioning subsystem for that operation. The positioning subsystem is said to be unavailable. (Note that the positioning subsystem may also be unavailable if a fault is detected within the receiver, cannot be mitigated and results in an alert to the client system.) This may result in the current operation being aborted or some adaptation of safety parameters in the way the operation is executed.
Figure 2 – The Stanford Diagram
In the analysis of positioning subsystem integrity performance, GNSS engineers make use of the Stanford diagram as illustrated in Fig.2. On this they plot a cloud of data from position updates obtained under real world test conditions. Studying this diagram may help in understanding the GNSS integrity concept. The axes are the PL and the PE, and the AL is marked by means of two straight lines parallel to the axes. A diagonal line divides the diagram into a safe zone and a Misleading Information (MI) zone. The AL lines create further zones, one of which represents Hazardous Misleading Information (HMI) where the PE exceeds the AL while the PL is below the AL. What we expect to see is that the MI Rate (MIR – i.e., the number of points plotted in the MI region compared to the total number of points) is consistent with the Target IR (TIR).
GET INVOLVED WITH THE CW JOURNAL & OTHER CW ACTIVITIES |
In aviation the nominal measurement errors are modelled by means of Gaussian overbounds which are Gaussian distributions that produce higher tail probability than the true (ie empirical) error probability distribution, which may be somewhat non-Gaussian. Using these error models, the error models for the three components of position can be derived and the PLs can be obtained based on these distributions assuming a linear process for the state computation. Since the overbounding state error distributions are also Gaussian, the calculations of the PLs based on the error variances is straightforward. In order to simplify the problem a snapshot least squares solution algorithm is used rather than the more usual iterative filtering employed in GNSS receivers.
Non-nominal errors are those that are not covered by the nominal error models. These are typically caused by hardware or software faults in the satellites and are typically referred to as faults. These faults must be monitored for with a sufficiently high detection probability that the probability of escaping faults is much lower than the target integrity risk. The monitoring can be via external messages from GNSS augmentation systems or via Receiver Autonomous Integrity Monitoring (RAIM). RAIM does not require additional infrastructure as the integrity monitoring is undertaken within the receiver. RAIM operates via a prescribed algorithm that involves monitoring the measurement residuals after the position calculation process. A key assumption that is critical to the validity of this algorithm is that simultaneous faults do not occur.
Of recent years many researchers have contributed to broadening the RAIM scheme for aviation, and this is coming to fruition in the form known generically as Advanced RAIM (ARAIM). One core idea is to use Multi-Hypothesis testing via Solution Separation (MHSS). That is, faults are hypothesized and the hypotheses are tested by running multiple solutions at each position update or ‘epoch’ excluding the hypothesized faulty measurements from solutions and comparing results from different solutions to decide whether a fault is present or not. ARAIM is a further advance aimed at monitoring for multiple simultaneous faults by hypothesizing combinations of faults to allow for the potential for simultaneous faults arising from concurrently using multiple GNSS constellations. It also accounts for varying satellite and constellation fault probabilities by adapting the error models based on parameters supplied from the ground via low bandwidth Integrity Support Messages.

The automotive environment is far harsher for GNSS than aviation. The error distributions are highly non-stationary and large outliers can occur frequently due to NLOS signals arriving at the antenna which can produce errors of arbitrary size and due to severe multipath distortion resulting from reflections from buildings, trees, bridges and so on which produces more constrained but still severe errors.
The required accuracy and corresponding ALs for automotive applications are much tighter than for aviation. Use cases range from roadway identification to lane identification where the latter would involve an AL of around 1m cross-track.
These factors lead to a requirement for an even more sophisticated high integrity automotive positioning. Carrier phase-based positioning is one way to approach the higher accuracy requirement, and. Kalman filtering is another. It is also clear that an even more advanced form of ARAIM will be needed since far more frequent faults or non-nominal errors caused by multipath and NLOS must be expected along with a much higher probability of simultaneous faults.
The main global research approach to the automotive GNSS integrity problem starts with adapting ARAIM for use with an Extended Kalman Filter (EKF) rather than with snapshot least squares as for aviation. The scheme again involves MHSS but, in order to achieve the required accuracy, now requires multiple Kalman filters running in parallel to test the fault hypotheses.
This automotive EKF-ARAIM concept involves the use of differential GNSS and carrier phase to improve accuracy. However, long baseline operation (large separation between rover and reference station) is needed to provide broad geographic coverage for huge numbers of vehicles and hence the ionospheric errors are not guaranteed to cancel especially during ionospheric disturbances. The residual ionospheric errors therefore represent integrity threats.
For this reason, typically, each Kalman filter uses iono-free combinations of measurements from two bands. The fact that the ionospheric delay is dispersive is exploited by forming linear combinations of measurements from the two bands so that the ionospheric delay is cancelled. Unfortunately, these combinations amplify the measurement noise and, especially, the multipath-induced error components. Furthermore, the carrier phase combinations do not exhibit integer phase ambiguities (i.e., there is no equivalent of a wavelength for the pseudo-measurement) and therefore normal high precision positioning using carrier phase measurements cannot be used. As a result there is a significant accuracy penalty for using the iono-free combinations.
Several further major impediments to very high integrity using EKF-ARAIM remain. Firstly, the EKF algorithm is a sequential filter and hence the evolution of the filter state must be taken into account when assessing the integrity at any given epoch. The PL computation must account for (1) the possible occurrence of faults in the history of measurements used by the filter and (2) the presence of temporally correlated errors. This requires a new overbounding scheme that is not yet proven. In the automotive environment, multipath errors are correlated over time. They represent slowly varying biases that can drag the filter away from the correct state. One solution to this problem is to periodically reset each filter while maintaining continuity by running a parallel filter that is reset at alternate times and switching between the two filters. Attempts are also made to model the time correlation of the measurements using an autoregressive model.
The measurement errors are also not Gaussian and yet the Kalman filter is based on Gaussian assumptions and relies on Gaussian stochastic models represented by measurement and state covariance matrices. The probability distributions of the errors exhibit fat tails and the calculation of the state covariances from the measurement covariances cannot represent these tails. The standard approach to this problem is to overbound the errors with Gaussian models as was done in the aviation case. However, in the automotive case, the measurement errors are typically far less Gaussian, and processing with a Kalman filter further complicates the problem. The key issue is that, until recently, it had not been proven that the resulting Gaussian state error models are guaranteed to overbound the state errors far out in the tails where the PLs lie.
Recently, a potentially viable solution to this problem has been found2. It turns out that overbounding the power spectral density of the measurement errors with a Gaussian model does guarantee overbounding of the state errors but only provided that the errors conform to a number of strict assumptions. So the problem that remains is to properly model the time correlation of the measurement errors with Gaussian models. That is complicated by the highly non-stationary nature of the measurement error statistics in the automotive environment and remains an area of very active research.
EKF-ARAIM is based on a set of assumptions that do not correspond to real world conditions and have to be worked around especially in relation to error overbounding. Each work-around has to be justified in order to prove that the PLs are correct at the extremely low IRs that are being sought for autonomous driving applications. This means that the verification and validation process of any EKF-ARAIM implementation must necessarily involve a huge amount of testing – at least given the current state of overbounding theory. To prove IR of 10-7/hr by test alone would require around 1 billion hours of driving in real world conditions representative of all the conditions under which the solution is expected to be used. This can be reduced, of course, by supplementing the real world testing with simulation, by using fault injection, hardware in the loop testing and so on. Nevertheless, the reliance on test for verification is necessarily huge for EKF-ARAIM. Another consequence of working around the initial false assumptions is that a very high level of conservatism is essential. Gaussian overbounding already demands a high degree of conservatism when fat-tailed distributions are to be overbounded and a great deal of further conservatism is needed to overcome time correlation and other issues that were discussed above.
DO YOU HAVE A VIEW ON THIS SUBJECT, OR RESPONSE YOU'D LIKE TO SHARE? |
In a bid to avoid most of these problems, the u-blox integrity research team decided to explore a completely different approach. The aim was to devise a theoretically rigorous solution that was amenable to analytic proof to a far greater degree than is EKF-ARAIM and to achieve far tighter bounds than are achieved with EKF-ARAIM. The result is what we call Single Epoch Position Bounding3. Rather than estimating position and then trying to bound the errors, we find the bounds on the position and then estimate the position as the mean of the bounds in each dimension. We do this using measurements from a single epoch with no filtering and then we propagate both the bounds and the position between the epochs using a safe technique as illustrated in Fig. 3. The propagation scheme (not described in this article) suffers minimal loss of accuracy during propagation and maintains rigorous bounds.
Figure 3 – Propagation Scheme for SEPB
Given a set of measurement error distributions, the posterior state distributions are obtained via Bayesian inference as illustrated in Fig. 4 and are numerically integrated to find the bounds appropriate to the required integrity risk. Both pseudorange and carrier phase measurements can be used and, when they are, very tight bounds are achieved. The numerical integration of the posterior distribution is undertaken using Markov Chain Monte Carlo (MCMC) techniques. Given that the posterior distribution is highly multi-modal when carrier phase measurements are employed and the required protection levels are very far out in the tails, the sampling of the posterior distribution must be undertaken with great care, but when all of this is done correctly, the performance achievable is far superior to that obtained using EKF-ARAIM.
Figure 4 – Bayesian Inference as Applied to SEPB
The measurement error models used in SEPB are constructed ahead of time, based on a massive measurement campaign using test vehicles as indicated in Fig. 5 and expansion of the campaign to larger but less elaborately instrumented fleets. A sophisticated batch post-processing scheme is used to derive very precise estimates of the true position trajectory and corresponding expected measurements. This is the ‘truth’ based on which the measurement residuals are extracted. These residuals are then analysed to derive error models that are conditioned on a small set of powerful measurement quality indicators (QIs). Parameterised error distributions are fitted to the empirical data as indicated in Fig. 6. The same QIs are used to reject measurements likely to have resulted from NLOS as well as whole epochs in which many measurements have very low quality. The impact of this on the tails of the measurement error distributions is remarkable as indicated in Fig. 7. This screening is important in order to eliminate the threat from NLOS and extreme multipath distortion.
Figure 5 – A Massive Measurement Campaign Underpins the SEPB Error Modelling
Figure 6 – Measurement Rejection Is Used to Exclude NLOS and Extreme Multipath
Figure 7 – Error Model Fitting
In the case of SEPB there are a few key assumptions underpinning the main algorithm. One is that the measurement error models used are accurate representations of the true error distributions. Another is that the sampling and integration of the posterior distribution is accurate. The verification of SEPB, therefore, is much more aimed at proving that these assumptions are met rather than trying to prove the integrity risk by test. The other key benefit of SEPB when carrier phase is used is very tight PLs, as shown in the results of Fig. 8 which were obtained from real world data across a wide range of road conditions. This is because SEPB takes full advantage of the integer carrier ambiguities but in a safe way – that is without fixing ambiguities. SEPB also avoids the high level of conservatism that arises from Gaussian overbounding.
The main disadvantage of SEPB is that it is very computationally intensive – at least when carrier phase measurements are used. On the other hand, to achieve very low integrity risk, EKF-ARAIM is also highly computationally intensive because a very large number of very low risk faults and combinations of faults become significant and have to be monitored via MHSS. In fact, many hundreds of Kalman filters would likely have to be run in parallel.
Figure 8 – SEPB-Based Horizontal Protection Limits
The race to provide integrity for GNSS-based positioning for automated driving is well and truly on. The requirements are daunting, need to achieve the same low integrity risk as for aviation but with a far harsher GNSS signal environment and requiring far higher levels of accuracy. Despite claims by some commercial competitors that they can offer such solutions, there is no public evidence that all of the many problems facing GNSS integrity research have been solved. However, significant progress has been made and there is light at the end of the tunnel.
The EKF-ARAIM approach draws on the pioneering work conducted in aviation by adapting ARAIM to use Kalman filters using dual band carrier phase techniques with iono-free measurement combinations. u-blox is heavily involved in EKF-ARAIM research but is also pursuing a completely different approach called SEPB. SEPB offers extremely tight protection levels but proof of integrity risk for a SEPB implementation is much easier with far less testing.
For moderate integrity risk, proof of risk for EKF-ARAIM is approachable and EKF-ARAIM is likely to be significantly less computationally demanding than SEPB. However, for very low integrity risk, SEPB can be verified far more readily than EKF-ARAIM while the computational demands may be quite similar. SEPB also provides much tighter PLs than EKF-ARAIM. For these reasons, we see room for both types of solution for future safe positioning in automotive applications, but also for robotics, machine control and drone operation beyond visual line of sight.
1Tyler G. R. Reid† et al, Localization Requirements for Autonomous Vehicles, SAE WCX, April 9-11 2019 Detroit
2Nikiforov I. From pseudorange overbounding to integrity risk overbounding. NAVIGATION. 2019;66:417–439. https://doi.org/10.1002/navi.303
3R. Bryant et al, Novel Snapshot Integrity Algorithm for Automotive Applications: Test Results Based on Real Data, ION PLANS 2020, April 20-23 2020 Oregon
Senior Director, Technology, Positioning at u-blox
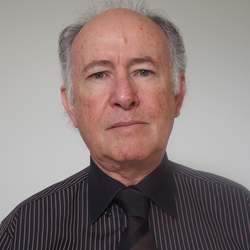